Introduction
AI (Artificial Intelligence) has been rapidly advancing, particularly in the field of language processing. Generative AI, in particular, has emerged as a powerful tool for creating human-like language. Understanding the intricacies of AI lingo is crucial for effective human interaction in the age of AI.
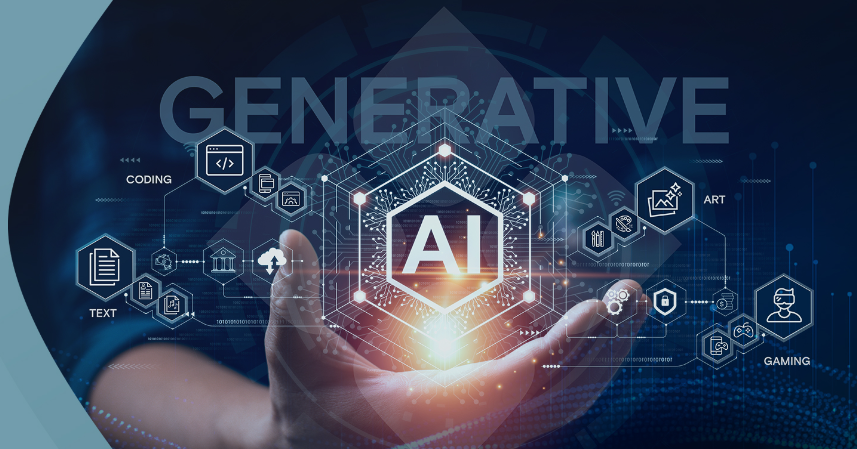
How Generative AI Speaks to Humans
This blog aims to explain the foundations of AI language, explore the learning journey of generative AI, delve into human-like AI speaking, highlight the significance and potential applications, address ethical considerations and future challenges, and conclude with key takeaways and encouragement for responsible AI usage.
The Rise of Generative AI
Generative AI has witnessed remarkable progress in recent years. It enables machines to generate human-like language, opening up a realm of possibilities for applications such as chatbots, language translation, and content generation. The ability of AI to comprehend and generate language akin to humans has revolutionized various industries, making it imperative to decode the AI lingo.
Importance of Understanding AI Lingo for Human Interaction
As AI becomes increasingly integrated into our daily lives, our ability to effectively interact with it becomes crucial. Understanding AI lingo allows us to communicate and collaborate seamlessly with machines, unleashing their full potential. Whether it’s conversing with a chatbot or working alongside an AI-powered assistant, comprehending AI language is essential for enhanced human-AI collaboration.
Unraveling the Foundations of AI-Language
Generative AI relies on a foundation of key concepts and techniques that enable machines to speak human-like language.
Fundamentals of Generative AI
- Definition and Evolution: Generative AI involves the use of neural networks and machine learning algorithms to generate language. It has evolved from rule-based systems to more sophisticated deep learning models.
- Key Components and Techniques: Generative AI encompasses various components, including language models, recurrent neural networks (RNNs), and transformers. Techniques such as attention mechanisms, beam search, and self-attention help improve language generation.
- Training Data and Neural Networks: Generative AI models require vast amounts of training data, which can include text from books, articles, and internet sources. Neural networks are trained on this data to learn patterns and generate language accordingly.
Natural Language Processing (NLP)
NLP plays a vital role in AI language understanding and generation.
- Understanding NLP in AI: NLP encompasses a range of techniques and algorithms that enable machines to understand and process human language. It involves tasks such as parsing, semantic analysis, and sentiment analysis.
- Parsing and Text Understanding: Parsing allows machines to understand the structure of a sentence or text, breaking it down into its components. This helps in extracting meaning and understanding context.
- Sentiment Analysis and Contextual Understanding: Sentiment analysis enables AI to discern the sentiment or emotional tone conveyed in the text. Contextual understanding involves comprehending the meanings of words and phrases based on the surrounding context, ensuring accurate language generation.
Cognitive Linguistics and Communication
Cognitive linguistics provides insights into human language and is instrumental in developing AI language models.
- Role of Cognitive Linguistics in AI-Language: Cognitive linguistics studies the mental processes involved in understanding and using language. It helps in building AI models that mimic human-like communication patterns.
- Conceptual Metaphors and Representations: Conceptual metaphors play a significant role in language understanding. By mapping abstract concepts onto more concrete ones, AI models can navigate complex language structures.
- Building Human-Like Communication Models: AI models can be trained to replicate human conversational patterns by incorporating cognitive-linguistic principles. This enables machines to speak in a way that humans can easily comprehend.
Generative AI: The Language Learning Journey
Generative AI goes through a learning journey to acquire language skills similar to humans.
Initial Training and Language Acquisition
- Pre-training on Datasets: Generative AI models are initially trained on vast datasets to learn patterns and acquire language knowledge. These datasets can include written text, transcriptions, and conversations.
- Unsupervised Learning and Self-Training: After the initial training, AI models continue learning through unsupervised techniques. They analyze vast amounts of text data and self-train to improve their language generation abilities.
- Fine-tuning with Human Interaction: To enhance their language skills, AI models are further fine-tuned using human interaction. Feedback from humans helps them adapt and improve their language generation capabilities.
Dialogue Systems and Chatbots
Dialogue systems and chatbots are examples of generative AI that facilitate interactive communication.
- Conversational Agents and their Mechanisms: Chatbots and dialogue systems are designed to engage in conversation with users. They utilize techniques such as intent recognition, dialogue state tracking, and response generation to provide meaningful and contextually relevant responses.
- Sequence-to-Sequence Models in Chatbots: Sequence-to-sequence models form the backbone of chatbot architectures. They generate responses based on input queries, taking into account context and conversation history.
- Reinforcement Learning for Dialogue Generation: Reinforcement learning is employed to train chatbots to generate more relevant and coherent responses. It involves providing rewards or reinforcement signals to the chatbot based on the quality of its generated responses.
Multimodel Communication and Understanding
Generative AI is evolving to understand and integrate multiple modes of communication.
- Integrating Text, Images, and Speech: AI models are being developed to process and generate language using various modalities such as text, images, and speech. This allows for more immersive and interactive communication experiences.
- Visual Question Answering and Image Captioning: AI models can be trained to answer questions about images or generate captions based on visual input. This facilitates better understanding and communication in multimodal contexts.
- Enhancing Interactive Communication Experiences: The integration of multimodal understanding enables AI to engage in more dynamic and nuanced conversations, providing users with a richer and more interactive communication experience.
Deep Dive into Human-Like AI Speaking
Generative AI has made significant strides in mimicking human-like language, offering creativity, contextual understanding, and emotional nuances.
Language Generation and Creativity
- Text Generation Models and Approaches: Generative AI models employ various techniques, including recurrent neural networks (RNNs), transformers, and GPT (Generative Pretrained Transformer) models, to generate text. These models can generate coherent and creative language.
- Generating Human-Like Stories and Narratives: AI models can be trained to generate compelling stories and narratives that closely resemble human storytelling. They learn narrative structures and plot development from vast datasets and generate unique and engaging narratives.
- Challenges and Ethical Considerations: While AI-generated stories can be impressive, there are concerns about plagiarism, intellectual property rights, and the potential for biases creeping into the generated content. Proper guidelines and ethical considerations need to be followed.
Contextual Understanding and Adaptability
- Context-Aware Language Models: AI models are being developed to understand and generate language in context. Techniques such as attention mechanisms and transformers enable them to take into account the context and generate more contextually relevant responses.
- Conversational Context and Coherence: AI models are trained to maintain coherence in conversations, understanding the preceding dialogue and generating responses that align with the ongoing conversation.
- Adapting to Different User Styles and Preferences: AI models can be customized to adapt to different user styles and preferences. They learn from user interactions and fine-tune their language generation capabilities to align with the user’s specific communication style.
Emotion and Sentiment in AI Communication
- Sentiment Analysis and Emotion Recognition: AI models can be trained to analyze and understand emotions conveyed in text. Sentiment analysis techniques enable them to determine the sentiment behind a piece of text, facilitating more emotionally responsive language generation.
- Incorporating Emotion into AI-Language: AI models are being developed to infuse emotion into their language generation. They can produce text that conveys different emotional tones, such as happiness, sadness, or excitement, based on the desired context.
- Ethical Implications and Responsible Use: The ability of AI to mimic emotions raises ethical concerns, as it can potentially be misused or manipulate human emotions. Responsible use and guidelines should be implemented to ensure the ethical and responsible deployment of AI language models.
The Significance and Potential Applications
AI language processing has significant implications and applications across various industries and sectors.
Advancements in AI Language Processing
- Recent Breakthroughs and Research Findings: Continuous advancements in AI language processing are being made. Recent breakthroughs, such as the development of GPT-3, have pushed the boundaries of generative AI language models.
- Improved Language Understanding and Generation: AI language models are continuously improving in their ability to understand and generate coherent and contextually relevant language. This paves the way for more natural and effective human-machine communication.
- Impacts on Various Industries and Sectors: AI language processing has far-reaching implications for industries such as customer service, healthcare, education, and content creation. Enhanced language understanding and generation can streamline processes and improve user experiences.
AI Language and Human-Machine Collaboration
- Enabling Effective Human-AI Interactions: As AI language processing improves, it enables more effective and seamless interactions between humans and machines. This can enhance productivity, facilitate decision-making, and augment human capabilities.
- AI as a Language Learning Assistant: AI language models have the potential to serve as language learning assistants, providing personalized tutoring, feedback, and language practice exercises to learners.
- Collaboration in Creative Content Generation: AI language models can collaborate with humans in generating creative content, such as writing articles, marketing materials, or even collaborating on artistic endeavors. This collaboration between humans and AI can result in innovative and captivating content.
Ethical Considerations and Future Challenges
- Bias and Fairness in AI Communication: AI language models are prone to biases present in the training data. Efforts are required to minimize biases and ensure fairness in language generation to prevent reinforcing societal prejudices.
- Preserving Privacy and Data Protection: As AI language models rely on vast amounts of data, privacy concerns arise. Proper data protection and privacy measures need to be in place to safeguard user information.
- Striking the Balance: Human Control and AI Autonomy: While progress in generative AI is exciting, there is a need to strike a balance between human control and AI autonomy. Guidelines and regulations should be established to ensure responsible and ethical use of AI language models.
Conclusion
Generative AI has transformed the way machines speak to humans. Understanding AI lingo is imperative in effectively interacting with AI systems. This article explored the foundations of AI language, the learning journey of generative AI, the nuances of human-like AI speaking, its significance and potential applications, as well as the ethical considerations and future challenges. By decoding the AI lingo, we can make the most of the powerful capabilities of generative AI while ensuring responsible usage.
FAQs
How does AI understand and generate human-like language?
AI understands and generates human-like language through training on vast datasets, utilizing techniques such as recurrent neural networks (RNNs) and transformers. These models learn patterns in the data and generate language accordingly, mimicking human-like communication.
Can AI understand different linguistic contexts and adapt accordingly?
Yes, AI can understand different linguistic contexts and adapt accordingly. Contextual understanding techniques, such as attention mechanisms and transformers, enable AI language models to generate more contextually relevant responses based on the conversation or text input.
What are the potential risks and ethical concerns associated with AI language generation?
Potential risks and ethical concerns include biases present in the training data, the manipulation of human emotions through AI-generated language, and the potential misuse of AI language models for misinformation or unethical practices. Guidelines and responsible use are crucial to address these concerns.
What are the limitations and challenges in developing AI systems that speak human?
Limitations and challenges include the potential for bias in language generation, ensuring coherent and contextually relevant responses, and preserving user privacy and data protection. Striking a balance between human control and AI autonomy is also a challenge.
How can AI language processing benefit different industries and domains?
AI language processing has wide-ranging applications across various industries and domains. It can enhance customer service, improve healthcare communication, facilitate language learning, streamline content creation processes, and augment human productivity in numerous fields.